Using Predictive Analytics To Forecast Future Trends, Identify Risks And Opportunities In Business
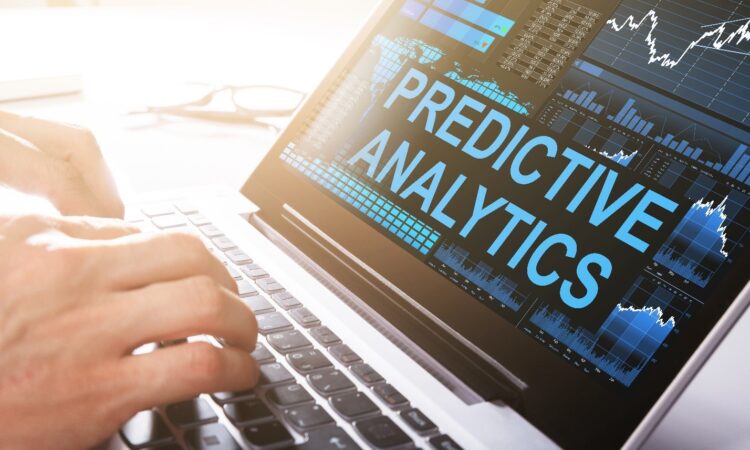
Imagine for a moment the first person on earth to start a business. For the first day, the first week, the first month, and even the first year, they had no data to go on. They could not predict what would happen based on past events. They had to “wing it” and hope they had the right goods and were targeting the right people. They no doubt also hoped their timing was right.
As time went on, they were able to organize themselves better using past data. They could, for example, tell which goods were fast movers in certain weather and which were not.
Humankind has used predictive analytics for as long as we have been trading. We have always observed what is happening around us and stored that information away to use for making decisions in the future.
There are several differences between how we use analytics today compared to ancient times. We have a lot more data to consider, many more variables and we have complex algorithms to make predictions. In some ways, it is easier, but it is also more complex.
What is predictive analytics?
It is the use of data, algorithms, and machine learning technologies to identify future outcomes using historical data. To put it simply, it is the use of past data to predict future trends that are used to shape strategic decisions. It can be used to predict the near future or the distant future.
In the past, data scientists made predictions manually. They would, for example, tabulate monthly sales for several years and try to identify trends that would guide future decisions.
New technologies have eliminated the need for this type of tedious analysis. Algorithms do all the work. With the right tools, a business can make all sorts of forecasts and identify risks.
The relevance of predictive analytics
Businesses that hope to survive in the current competitive environment must strive to make the best possible predictions using the data at hand.
According to a report by Business Wire, global spending on data analytics reached $215 billion in 2021 and is expected to grow by about 13% by 2025. This is an indication that businesses take analytics seriously and are willing to spend big bucks to make predictions.
They are also willing to pay well for people with the right analytics skills. If you enjoy data, mathematical modeling, and making predictions, you can earn a six-figure income with the right qualifications.
An online business analytics masters degree, for example, equips students with the data analysis skills that American businesses are looking for. The online course by St Bonaventure University teaches students how to make ethical decisions using the data at hand, and imparts a deeper understanding of global business through data.
If this appeals to you, it is a good idea to familiarize yourself with predictive analysis. What is it, and why is it important? How is it used to forecast the future of business? How can it be applied to identify risks and opportunities?
Why do businesses need predictive analytics?
Why should a business spend money doing statistical modeling and analysis? Owners and managers can read newspaper forecasts and watch the news to find out what experts think about the future.
Unfortunately, that isn’t enough. Every business is unique, and a forecast that applies to one may not be relevant to another. It is important to conduct predictive analytics for the following reasons:
- It is a smart way to optimize marketing campaigns. An analytical model will tell you how customers respond to a certain product, what sales look like at different times of the year, and what demographics react to different marketing campaigns. Businesses can use this information to create more targeted campaigns for a healthier bottom line.
- Analytics provide a blueprint to improve operations. When you analyze data, you can see inefficiencies and bottlenecks within the business. You can, for example, identify delays in the sales funnel and use the information to streamline operations.
- Analytics is the best way to avoid risk. It can be as simple as assessing a buyer’s ability to pay or deciding where to source raw materials. By looking at a potential customer’s credit history, for example, you can see whether or not they will settle your bill quickly. You can also look at past data to determine whether your suppliers will deliver raw materials on time and at a competitive price.
- Predictive analysis is a good fraud detection tool. It highlights abnormalities and allows businesses to take corrective action before it is too late.
How are businesses using predictive analysis?
There are numerous ways that predictive analysis helps businesses.
Uncovering hidden patterns
The world has become quite complex. Economies react to the smallest changes, customer preferences can be affected by a midnight tweet from a company CEO, suppliers can increase prices based on what they think will happen tomorrow, and an online trend can make or break a business.
Those who uncover these patterns early enough tend to survive the resulting turmoil. Businesses that take a reactionary position do not excel.
Predictive analytics are excellent for detecting hidden patterns. Feeding data into the right software reveals connections that may not be obvious.
It is great for enhancing customer retention
Do you know what your customers like? Do you know what products they spend money on and when they buy them? Who buys what, and how much are they prepared to spend? How do customers react to campaigns at different times of the year?
These are all questions that can be answered with predictive analytics models. By feeding previous sales data into forecasting software, you can tell with some degree of accuracy what will happen in the future.
Predictive analysis improves cross-selling opportunities
Cross-selling is a huge source of revenue for many businesses. It means selling a different product to an existing customer. Analyzing buying trends tells businesses what customers are buying, and they can identify other products likely to appeal to them.
Amazon is great at cross-selling. When a user buys a product, the company uses software to scan their product range for related products that may be of interest and sends them adverts. Odds are they will see something else they like and make a purchase.
Many businesses miss out on revenue opportunities because they do not cross-sell. Predictive modeling software can help them identify which items are likely to sell based on previous purchases, and they can create personalized campaigns for their customers.
Predictive analytics helps with business alignment
Business alignment means that all the elements of a company work together for the best results. Human resources and company assets are put to the best use for maximum productivity.
Predictive analytics identifies bottlenecks and inefficiencies. By looking at past data, for example, you may realize that shipping to certain parts of the country took longer than it should during a certain period. Finding out why helps you reorganize and eliminate the problem.
Businesses can identify risks and detect fraud
Today’s business world is fraught with risk, and the only way to navigate it is with good data and analytics models. A good example is climate change. A predictive model can tell you whether you should expect a flood or a drought, and you can factor that information into your plans.
Detecting fraud with predictive models is easy. All you need to look for is unusual trends and find out why they are happening.
Predictive analysis helps identify potential risks and assess their impact so that they can take corrective measures in time.
Companies can improve customer satisfaction and retention
Because you use predictive analytics to determine customer preferences, you give them what they want. A satisfied customer is likely to buy again. Not only does this improve the bottom line, but it also helps with brand engagement.
Businesses can improve customer segmentation
Segmentation is everything if you want to run a successful sales campaign. Analytics helps businesses focus on getting the right products to the right customers at the right time.
Data can be used for predictive maintenance
Predictive maintenance is vital in machinery-heavy businesses. For production lines to work smoothly, equipment must be functional at all times.
Predictive analytics allows companies to schedule maintenance. By looking at past data, production managers can tell when a piece of equipment will slow down, and they can make appropriate plans.
Past data can tell a production manager how many runs a machine can make before it needs maintenance or how many pieces it can produce before some parts need replacement.
Instead of waiting for a breakdown to take action, the manager can be proactive, ensuring that machines run at peak performance at all times.
Real-life examples of analytics at work
Banking
Banking deals with huge amounts of data and money and has long relied on predictive analysis to make sound operational decisions.
By looking at past data, banks and other financial institutions can detect fraud, understand how they will be impacted by market shifts, maximize cross-selling opportunities, and improve customer retention.
The simplest example of banks using predictive modeling is when a customer applies for a loan.
By looking at their credit history, a bank can see whether or not they are good at paying off debts. If the customer has a poor relationship with credit, it will be revealed by past data, and the bank can decline the loan, thus avoiding future difficulties.
Oil and gas
These are arguably the two most important commodities in the world, and their price is set using predictive analysis. By looking at past data, for example, countries that supply oil and gas can decide on how many barrels to release into the market every day and how much each barrel will cost.
Utilities
Utility companies in America use past data to determine how much power and water they should supply to different states. By looking at recent climate trends, for example, they know when power usage spikes and can plan accordingly.
California is a case in point. It has been affected by severe drought in the last decade or so. During that time, utility companies have kept the state going by purchasing water from the Colorado Aqueduct.
Previous data allows them to predict whether or not it will rain and how much rain the state will receive. They use this information to decide how much water they should buy to satisfy homes and businesses across the state.
Healthcare
This is one of the best examples of using past data to predict future trends. The industry can use historical trends to identify and plan for the country’s healthcare needs.
Many do not know it, but long before the pandemic occurred, experts had been predicting for years that it was nigh. They warned governments to prepare. Unfortunately, not many did, and eventually, when COVID-19 struck, they struggled to provide basic care.
Another excellent example of using statistical analytics in healthcare is planning for baby boomers. Most of them are now elderly and suffer from chronic illnesses. Despite warnings from experts, the government did not prepare adequately, and the country now has to deal with a shortage of nurses.
Analytics is also used every year to predict seasonal illnesses like the flu. Hospitals and other healthcare facilities can make adequate plans for vaccinations, bed space, and personnel.
Retail
Retail companies use predictive analysis to plan for merchandise, determine prices, create sales campaigns, and optimize cross-selling.
By looking at past data, a retail company gets a fairly clear idea of who their customers are, how much they are willing to spend for different products, when those products are most likely to sell, and which sales campaigns are most effective.
Online retailers are especially reliant on this data. Just look at the way Amazon uses your data, for example. It knows exactly when to have sales, how much it should price different items to ensure that they move, and even what products it should market in different countries.
Governments
Governments are just as reliant on predictive analysis as businesses. It is a smart way to allocate resources. A good example is infrastructure. Using data modeling and statistical analysis, the United States government can predict when infrastructure will need an overhaul and plan accordingly.
The government uses statistical models to plan for immigration, healthcare, trade, education, jobs, utilities, and more.
Manufacturing
After you complete an online business analytics master’s degree, you may be employed by a manufacturing company. You will be expected to use your knowledge to streamline operations and plan accordingly.
Using past data, manufacturing companies identify factors that affect production. Weather predictions, for example, are critical for these businesses.
Market trends also determine output levels, and even things like warranty levels can be predicted with customer data.
Making the most of predictive analytics
Predictive analytics is not a magic bullet. Businesses must understand how best to use it if they hope to gain from it. There are certain things that managers must avoid if they want analytics to work:
They should not treat it like an objective
An objective is defined by setting goals and timelines. Predictive analytics doesn’t quite work like this.
If you want to use it successfully, you must learn to find value in the data you collect every day.
It must be a core part of your business. Help employees to understand that the information they collect from each transaction or interaction contributes to future decisions.
They must get the right people on the team
A common mistake that companies make is to buy an analytics software and then assemble a team around it. This often does not work. Rather than buy software and then put together an analytics team, identify the problems that your business faces and be clear about how you can solve them using analytics.
They should ensure all departments are represented
A data analytics team will only work if they have data from every department. Teams should have cross-company representation.
They must define what data is important
You can decide what data you should collect by identifying issues that can be solved using predictive analytics. Conduct a data audit once or twice a year to determine whether you are on the right track.
Managers should keep up with the latest in the industry
Analytics software is improving all the time. Developments in AI allow companies to do more with data than they were able to before. Companies that rely on outdated technologies are likely to be left behind by their competitors. Data experts and statisticians should aim to keep up with industry developments.
If a company decides to move to new software, it is the role of management to ensure that everyone understands how to use it and the value it adds to the business. This is called getting employee buy-in, which can be the difference between success and failure.
Conclusion
In the uncertain business environment that we live in today, it can often feel like we are operating blindly. Predictive data analysis helps counter this. Investment in predictive software and personnel can help businesses predict the future with a measure of certainty, avoid risks and take advantage of opportunities promptly.